How Artificial Intelligence Can Improve Our Understanding of the Genes Associated with Endometriosis.
Jun 5, 2018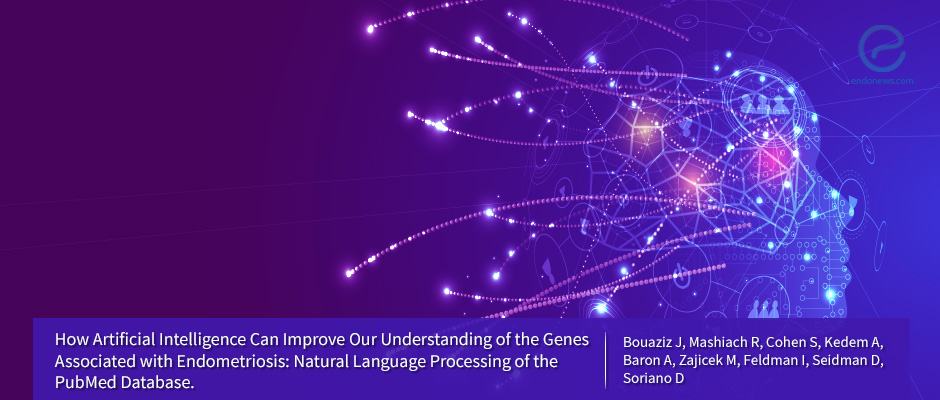
Dr. Bouaziz group reports that artificial intelligence can help us understand gene networks and pathways, candidate genes, and novel genes in endometriosis.
Key Points
Highlight:
- The use of artificial intelligence through text mining combined with bioinformatics techniques can help us understand gene networks and pathways, candidate genes, and novel genes.
- The Dr. Bouaziz group from Israel found that the top 6 genes associated with endometriosis are CDKNB2, MAPK1, WNT4, ILA, AKT1, and KRAS, which agree with those from microarray and genome-wide analysis studies.
Background:
- The cause of endometriosis remains largely unknown.
- Numerous genes have been studied and proposed to help explain its pathogenesis, but a large number of these candidate genes has made functional validation through experimental methodologies nearly impossible.
- Computational methods could provide a useful alternative for prioritizing those most likely to be susceptibility genes.
- The Dr. Bouaziz group from Ichilov Hospital in Israel analyzed the genes involved in the pathogenesis, development, and progression of endometriosis using artificial intelligence.
Key points:
- The text mining (TM) data extraction was based on natural language processing. The PubMed database of “endometriosis and genes” was used as a source for the TM process.
- A total of 203 genes reached the statistical significance threshold and were used to generate the word cloud, the network, and the pathway enrichment.
- The top 6 genes associated with endometriosis found in this study are CDKNB2, MAPK1, WNT4, ILA, AKT1, and KRAS, which agree with those from microarray and genome-wide analysis studies, a highly encouraging outcome.
Conclusion:
- The use of artificial intelligence through TM combined with bioinformatics techniques can help us understand gene networks and pathways, candidate genes, and novel genes.
- The rapid improvement in algorithm and machine learning efficiency is of great interest in helping researchers focus on the relevant candidates and novel genes for endometriosis before performing in vivo studies or microarrays.
Lay Summary
Endometriosis is a disease characterized by the development of endometrial tissue outside the uterus. A number of studies have shown that endometriosis could have a genetic basis and numerous genes have been studied and proposed to help explain its pathogenesis. However, the large number of these candidate genes has made functional validation through experimental methodologies nearly impossible.
Artificial intelligence using text mining (TM) technology has recently been implemented, enabling the automated retrieval of all candidate genes for a specific disease from all published experimental results. In a recent paper, Dr. Bouaziz group from Ichilov Hospital in Israel analyzed the results of the TM analysis of PubMed literature on genes involved in the pathogenesis of endometriosis. They also used bioinformatics to analyze the functions, pathways, and networks of relevant candidate genes, seed genes, and novel genes.
The TM data extraction was based on natural language processing. The PubMed database which contains “endometriosis and genes” was used as a source of publications for the TM process. The titles and abstracts of these articles (a total of 999 articles from 1969 to 2017) were retrieved and processed through a computational pipeline through text mining, which yielded a total of 724 genes. The text mining results were then filtered by Phenolyzer software. A total of 203 genes reached the statistical significance threshold and were used to generate the word cloud, the network, and the pathway enrichment. The top 6 genes associated with endometriosis found in this study are CDKNB2, MAPK1, WNT4, ILA, AKT1, and KRAS, which agree with those from microarray and genome-wide analysis studies, a highly encouraging outcome.
In conclusion, "The use of artificial intelligence through TM combined with bioinformatics techniques can help us understand gene networks and pathways, candidate genes, and novel genes. The rapid improvement in algorithm and machine learning efficiency is of great interest in helping researchers focus on the relevant candidates and novel genes for endometriosis before performing in vivo studies or microarrays" Dr. Bouaziz says.
Research Source: https://www.ncbi.nlm.nih.gov/pubmed/29750165
Artificial Intelligence Endometriosis Natural Language Processing PubMed Database