Genetic prediction models for endometriosis diagnosis
May 16, 2022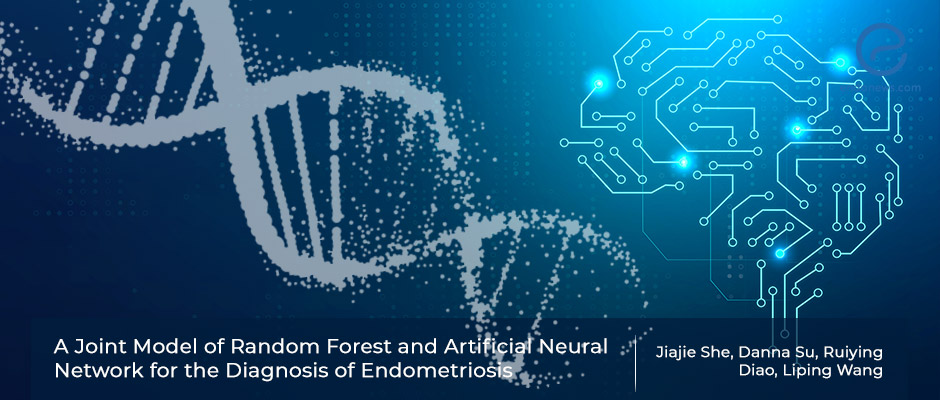
Artificial intelligence meets genetics to serve endometriosis diagnosis
Key Points
Highlights:
- Seven genes venerating 3 mechanisms have been identified with a prediction rate of 70% for endometriosis diagnosis.
Importance:
- There are so many genetic studies to identify the "extraordinary" gene that reveals endometriosis, but none have been found up to day.
- The present study conducted an artificial intelligence model to gather all results together to define a prediction model.
What's done here:
- The researchers used public datasets in Gene Expression Omnibus and Array-Express database.
- Differential expression analysis was conducted on 77 endometriosis and 71 normal samples.
- From the protein-protein interaction network, only the interactions with “high confidence" were selected.
- Statistical evaluation for sensitivity and specificity was performed.
Key Results:
- More than two thousand five hundred differently expressed genes related to endometriosis were identified in the GSE51981.
- Based on the random forest classifier, seven important differentially expressed gene candidates, with high accuracy prediction rates were chosen, which are COMT, NAA16, CCDC22, EIF3E, AHI1, DMXL2, and CISD3.
- AHI1, DMXL2, and CISD3 have been found to be related to hub genes of endometriosis meaning more than 90% of reactions with other expressed genes in the diagnostic dataset:
- The authors explain that these three genes might participate in the pathogenesis of endometriosis through oxidative stress, epithelial-mesenchymal transition with the activation of the Notch signaling pathway, and mitochondrial homeostasis.
Limitations:
- The study has analyzed the mRNA profiling data of 3 different datasets with a high-reliability index. However, the expression of those genes on protein level should be examined to cover posttranslational modifications and effects of differently expressed genes on real life.
Lay Summary
There are many studies trying to find a mysterious gene that would solve all the mysteries of endometriosis, yet none have been identified up to now.
In the study conducted by She et al, the authors revealed an R system modeling using artificial intelligence and literature-based gene data sets that covers a comparison of healthy and endometriosis diagnosed patients’ genes aiming to define a diagnostic system.
The researchers downloaded public datasets to the R package to obtain an annalistic profile of the literature and applied principal component analysis for comparison. In the protein-protein interaction network, the minimum required interaction score was set to a high degree of confidence to be used in diagnostic modeling. The sensitivity and specificity of the tests were statistically evaluated.
GSE6364 dataset that contains 21 endometriosis and 21 control samples was used as the training set to construct the artificial neural network model. More than 2,550 endometriosis-related differently expressed genes have been identified in the GSE51981 dataset. Based on the random forest classifier, seven important differentially expressed gene candidates, namely COMT, NAA16, CCDC22, EIF3E, AHI1, DMXL2, and CISD3 with a high accuracy prediction rate were identified.
Among those, AHI1, DMXL2, and CISD3 were found to be related to hub genes of endometriosis, meaning that these genes had more than 90% of reactions with other genes in the diagnostic dataset. The authors explained that these three genes might participate in the pathogenesis of endometriosis through oxidative stress, epithelial-mesenchymal transition by the activation of the Notch signaling pathway, and mitochondrial homeostasis.
The study only covers RNA results, and protein expression data were not evaluated, but still, the results promise a favorable future for the use of artificial intelligence in endometriosis diagnosis. The details of the study can be found in the recent issue of the journal "Frontiers in Genetics".
Research Source: https://pubmed.ncbi.nlm.nih.gov/35350240/
artificial intelligence genetic diagnosis