A self-diagnostic tool that predicts the likelihood of endometriosis
Apr 25, 2023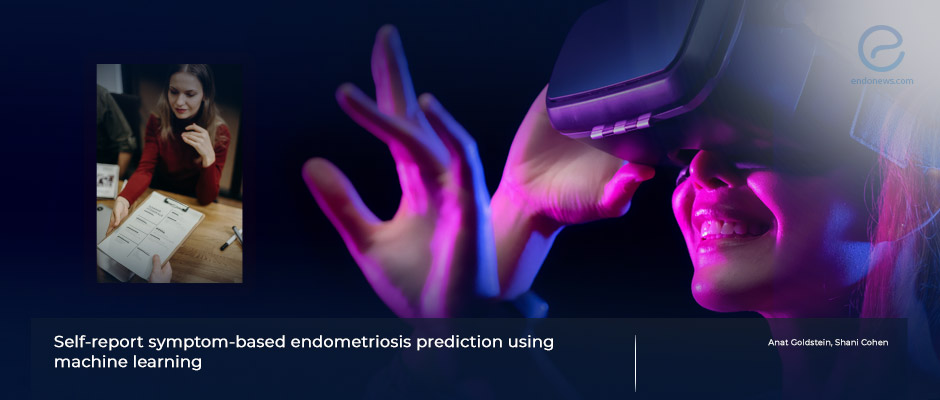
Artificial intelligence is on the stage for endometriosis self-diagnosis.
Key Points
Highlights:
- The study developed a machine learning model to predict endometriosis based on self-reported symptoms.
Importance:
- Machine learning can potentially improve the diagnosis and management of endometriosis, leading to early diagnosis.
What's done here:
- The authors collected data from women who completed a survey of symptoms related to endometriosis and underwent diagnostic laparoscopy to confirm the presence or absence of the disease.
- The data was used to train a machine learning model to predict the presence of endometriosis based on self-reported symptoms alone.
- The model was evaluated on a separate set of women who completed the same symptom survey and underwent laparoscopy for diagnosis.
Key Results:
- The model was trained on data from 1,592 women and evaluated on a separate set of 398 women.
- The machine learning model had a high accuracy in predicting the presence of endometriosis based on self-reported symptoms alone.
- The model was able to identify specific symptoms that were most predictive of the disease, including dyspareunia, dysmenorrhea, and chronic pelvic pain.
- The study provides a promising example of the potential of machine learning to improve the diagnosis and management of endometriosis.
Limitations:
- Additional research is needed to validate the model's performance in larger and more diverse populations.
- The study was limited to self-reported symptoms and did not include other clinical or demographic variables that may be important predictors of endometriosis.
Lay Summary
Early diagnosis and treatment of endometriosis are crucial for preventing these negative outcomes, but the disease is often difficult to diagnose due to a lack of specific symptoms and reliable diagnostic tests.
Goldstein et al. aimed to develop a machine-learning model that could predict the presence of endometriosis in women based on self-reported symptoms alone. The authors collected data from 1,592 women who completed a survey of symptoms related to endometriosis and underwent diagnostic laparoscopy to confirm the presence or absence of the disease.
The survey included questions about pain during intercourse, menstrual pain, chronic pelvic pain, and other symptoms commonly associated with endometriosis. The authors used this data to train a machine-learning model using a variety of algorithms and feature selection methods. They then evaluated the model's performance on a separate set of 398 women who completed the same symptom survey and underwent laparoscopy for diagnosis.
The results showed that the machine learning model had a high accuracy in predicting the presence of endometriosis based on self-reported symptoms alone. The model also identified the specific symptoms that were most predictive of the disease, including dyspareunia, dysmenorrhea, and chronic pelvic pain.
The authors suggest that this approach could be useful in identifying women who are at risk for endometriosis and may benefit from further diagnostic testing or early intervention. However, they also note that additional research is needed to validate the model's performance in larger and more diverse populations. Overall, the study provides a promising example of the potential of machine learning to improve the diagnosis and management of endometriosis.
Research Source: https://pubmed.ncbi.nlm.nih.gov/37016132/
AI endometriosis diagnosis